Summary
人口知能(AI)は創薬における長年の問題を解決できる技術として注目を浴びています。この調査レポートは、最適化や化学合成計画などのAI技術の開発企業、ならびに用途に着目しています。
主な掲載内容(目次より抜粋)
-
全体概要および結論
-
はじめに
-
創薬プロセスにおける人口知能
-
市場の状況
-
業界概要
Report Details
The development of pharmaceutical drugs is a long and costly process. Companies in the pharmaceutical and biotechnology industries typically spend more than $1 billion to bring a drug to market, in a process that often lasts over 10-15 years. Moreover, the drug development process is very risky - up to 90% of drug candidates are eventually dropped during the process due to issues such as safety and efficacy, resulting in massive losses for companies. Any technology that can contribute significantly to solving any of these three pain points of the drug development process will quickly grow into a multibillion-dollar industry.
One such technology that has emerged over the past few years is the use of artificial intelligence (AI), particularly machine learning (ML) and deep learning (DL) algorithms, to improve the drug discovery process. In this early stage of the drug development process, compounds of interest are identified and optimized to have drug-like properties before they are tested in animals, and later, humans. While computers have been used in aiding pharmaceutical R&D for many decades and even AI itself has been applied for more than 10 years, it has only recently started to gather momentum. Case in point - over 80% of funding for AI in drug discovery has been raised in the past 3 years, with investment over 2020, during the height of the COVID-19 pandemic, more than that of 2018 and 2019 combined.
Why apply AI in drug discovery?
Companies commercializing AI drug discovery platforms and AI-discovered drugs have shown that the use of algorithms can accelerate a multi-year process to a matter of months. This drastic decrease in development time along with the reduction of the number of compounds that need to be synthesized for laboratory testing, allows for significant cost savings, addressing two core issues of pharmaceutical R&D. While AI drug discovery companies have not necessarily proven that their technologies can bring a drug to market (i.e., successfully pass clinical trials) with higher rates of success than traditional drug discovery methods, the accelerated timelines and potential for cost savings are compelling enough for pharmaceutical companies across the world to either invest internally to develop their own AI capabilities, and to partner up with AI companies in billion-dollar deals.
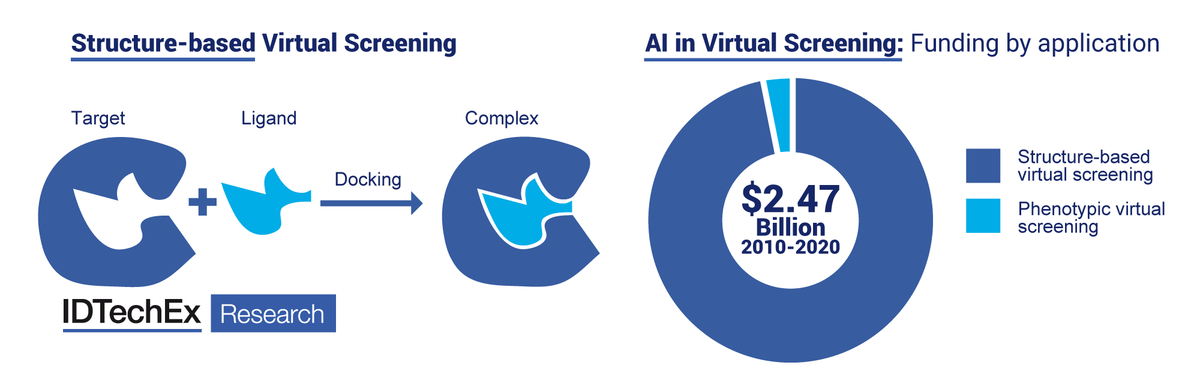
Structure-based virtual screening identifies molecules (ligands) that are predicted to bind to a biological structure (target). Structure-based virtual screening is the leading form of AI in drug discovery being funded today. Source: IDTechEx Research
How is AI applied in drug discovery?
In this report, IDTechEx have focused on the areas of virtual screening and de novo drug discovery as two aspects of drug discovery in which significant activity is occurring. Specific applications such as structure-based virtual screening are receiving significant attention, but it is not yet fully clear which aspect of AI in drug discovery will have the most impact in the future. While structure-based virtual screening is enabled by ready availability of structural data on which to apply AI algorithms, the complexity of biological systems means that structure and fit of compounds do not indicate a compound's safety and efficacy as a drug. Technologies such as phenotypic virtual screening and de novo drug discovery may hold more promise for first-in-class and even multi-target drugs, and all aspects will be supported by the application of AI in the prediction and optimization of a compound's properties.
What's in the report?
This report covers four aspects of the drug discovery process:
-
Virtual screening, including structure-based virtual screening, ligand-based virtual screening, and phenotypic virtual screening
-
De novo drug design
-
Lead optimization (predicting and optimizing compound properties)
-
Chemical synthesis planning
Within each aspect of the drug discovery process discussed, IDTechEx provides:
-
Key players
-
Funding (including breakdown by application and drug type)
-
Technologies
-
Company profiles (including interviews)
-
Progress of candidates to market
-
Software capabilities
-
Technology readiness
ページTOPに戻る
Table of Contents
1. |
EXECUTIVE SUMMARY |
1.1. |
Report Scope |
1.2. |
Report Scope: Drug Discovery |
1.3. |
Challenges in the Drug Discovery Process |
1.4. |
AI in Drug Discovery: Why Now? |
1.5. |
Drivers & Constraints of AI in Drug Discovery |
1.6. |
AI in Virtual Screening |
1.7. |
AI in Virtual Screening: Key Players |
1.8. |
AI in Virtual Screening: Conclusions |
1.9. |
AI in De Novo Drug Design |
1.10. |
AI in De Novo Drug Design: Key players |
1.11. |
AI in De Novo Drug Design: Conclusions |
1.12. |
AI in Lead Optimization |
1.13. |
AI in Chemical Synthesis Planning |
1.14. |
Funding in AI in Drug Discovery |
1.15. |
AI in Drug Discovery: Business Models |
1.16. |
AI in Drug Discovery Market Landscape: By Geography |
1.17. |
AI in Drug Discovery Market Landscape: By Application |
1.18. |
AI in Drug Discovery: Market Outlook |
1.19. |
Conclusions |
2. |
INTRODUCTION |
2.1. |
Report Scope |
2.2. |
The Drug Development Process |
2.3. |
Report Scope: Drug Discovery |
2.4. |
Key Terminology: Targets and Ligands |
2.5. |
Targets and Ligands: Lock and Key Analogy |
2.6. |
Challenges in the Drug Discovery Process |
2.7. |
Drug Discovery is Expensive |
2.8. |
History of AI in Drug Discovery |
2.9. |
AI in Drug Discovery: Why Now? |
2.10. |
Benefits of AI in Drug Discovery |
2.11. |
Drivers & Constraints of AI in Drug Discovery |
3. |
AI IN DRUG DISCOVERY |
3.1.1. |
What is Artificial Intelligence? |
3.1.2. |
AI, ML & DL in Drug Discovery |
3.1.3. |
AI Methods in Drug Discovery |
3.1.4. |
Applicability and Predictive Capabilities of Key AI Algorithms |
3.1.5. |
Constructing an AI Model: Which Algorithms to Use? |
3.1.6. |
How are Compound Structures Encoded into an AI Model? |
3.1.7. |
Molecular Fingerprints |
3.1.8. |
Simplified Molecular Input Line Entry Specification (SMILES) |
3.2. |
AI in Virtual Screening |
3.2.1. |
AI in Virtual Screening |
3.2.2. |
AI in Virtual Screening: Key Players |
3.2.3. |
AI in Virtual Screening: Funding |
3.2.4. |
AI in Virtual Screening: By Application and Drug Type |
3.2.5. |
Structure-Based Virtual Screening |
3.2.6. |
Recursion Pharmaceuticals |
3.2.7. |
Atomwise |
3.2.8. |
Micar Innovation |
3.2.9. |
TwoXAR |
3.2.10. |
Ligand-Based Virtual Screening |
3.2.11. |
Tencent |
3.2.12. |
Phenotypic Virtual Screening |
3.2.13. |
e-Therapeutics |
3.2.14. |
AI in Virtual Screening: Progress from Lab to Bedside |
3.2.15. |
AI for Virtual Screening: Clinical Trials |
3.2.16. |
AI for Virtual Screening: Partnerships |
3.2.17. |
AI in Virtual Screening: Software Capabilities |
3.2.18. |
AI in Virtual Screening: Technology Readiness |
3.2.19. |
AI in Virtual Screening: Conclusions |
3.3. |
Phenotypic Screening: AI for Cell Sorting and Classification |
3.3.1. |
Image Recognition AI |
3.3.2. |
Classification of Phenotypic HTS Results |
3.4. |
AI in De Novo Drug Design |
3.4.1. |
AI in De Novo Drug Design |
3.4.2. |
AI in De Novo Drug Design: Key players |
3.4.3. |
AI in De Novo Drug Design: Funding |
3.4.4. |
AI in De Novo Drug Design: By Drug Type |
3.4.5. |
How does AI-driven De Novo Drug Design Work? |
3.4.6. |
DMTA Cycles Must be Reduced |
3.4.7. |
How does AI-driven De Novo Drug Design Work? |
3.4.8. |
IBM Research Zurich |
3.4.9. |
Insilico Medicine |
3.4.10. |
Exscientia |
3.4.11. |
CaroCure |
3.4.12. |
Aqemia |
3.4.13. |
GlamorousAI |
3.4.14. |
AstraZeneca |
3.4.15. |
Arzeda |
3.4.16. |
BenevolentAI |
3.4.17. |
AI in De Novo Drug Design: Partnerships |
3.4.18. |
AI in De Novo Drug Design: Progress from Lab to Bedside |
3.4.19. |
AI in De Novo Drug Design: Software Capabilities |
3.4.20. |
AI in De Novo Drug Design: Software Capabilities |
3.4.21. |
AI in De Novo Drug Design: Technology Readiness |
3.4.22. |
AI in De Novo Drug Design: Conclusions |
3.5. |
AI in Lead Optimization |
3.5.1. |
AI in Lead Optimization |
3.5.2. |
History of Lead Optimization |
3.5.3. |
Key Properties and AI Algorithms |
3.5.4. |
Predictive Capabilities of Key AI Algorithms |
3.5.5. |
AI in Lead Optimisation: Process |
3.5.6. |
Quantitative Structure-Activity Relationship Models |
3.5.7. |
Intellegens |
3.5.8. |
PEACCEL |
3.5.9. |
ProteinQure |
3.5.10. |
Iktos |
3.5.11. |
Molomics |
3.5.12. |
Denovicon Therapeutics |
3.5.13. |
XtalPi |
3.5.14. |
Peptone |
3.5.15. |
GlaxoSmithKline |
3.5.16. |
AI in Lead Optimization: Software Capabilities |
3.5.17. |
ページTOPに戻る
IDTechEx社の半導体、コンピュータ、AI - Semiconductors, Computing, AI分野での最新刊レポート
本レポートと同じKEY WORD()の最新刊レポート
- 本レポートと同じKEY WORDの最新刊レポートはありません。
よくあるご質問
IDTechEx社はどのような調査会社ですか?
IDTechExはセンサ技術や3D印刷、電気自動車などの先端技術・材料市場を対象に広範かつ詳細な調査を行っています。データリソースはIDTechExの調査レポートおよび委託調査(個別調査)を取り扱う日... もっと見る
調査レポートの納品までの日数はどの程度ですか?
在庫のあるものは速納となりますが、平均的には 3-4日と見て下さい。
但し、一部の調査レポートでは、発注を受けた段階で内容更新をして納品をする場合もあります。
発注をする前のお問合せをお願いします。
注文の手続きはどのようになっていますか?
1)お客様からの御問い合わせをいただきます。
2)見積書やサンプルの提示をいたします。
3)お客様指定、もしくは弊社の発注書をメール添付にて発送してください。
4)データリソース社からレポート発行元の調査会社へ納品手配します。
5) 調査会社からお客様へ納品されます。最近は、pdfにてのメール納品が大半です。
お支払方法の方法はどのようになっていますか?
納品と同時にデータリソース社よりお客様へ請求書(必要に応じて納品書も)を発送いたします。
お客様よりデータリソース社へ(通常は円払い)の御振り込みをお願いします。
請求書は、納品日の日付で発行しますので、翌月最終営業日までの当社指定口座への振込みをお願いします。振込み手数料は御社負担にてお願いします。
お客様の御支払い条件が60日以上の場合は御相談ください。
尚、初めてのお取引先や個人の場合、前払いをお願いすることもあります。ご了承のほど、お願いします。
データリソース社はどのような会社ですか?
当社は、世界各国の主要調査会社・レポート出版社と提携し、世界各国の市場調査レポートや技術動向レポートなどを日本国内の企業・公官庁及び教育研究機関に提供しております。
世界各国の「市場・技術・法規制などの」実情を調査・収集される時には、データリソース社にご相談ください。
お客様の御要望にあったデータや情報を抽出する為のレポート紹介や調査のアドバイスも致します。
|
|